ANALOGICAL REASONING
A major focus of the Reasoning Lab is on the role of analogy in thinking and learning. Holyoak and his colleagues developed a method for experimentally studying the use of cross-domain analogies in problem solving, suitable for use with people ranging in age from preschool children to adults. Our early research showed that different types of similarity have differential impact on retrieval versus mapping, and that analogical transfer enhances learning of new abstract concepts. Other work demonstrated how analogy can be used to teach complex scientific topics by allowing transfer across different knowledge domains, and how analogy can be used as a powerful tool for persuasion in areas such as foreign policy. A detailed neural-network model of relational thought, LISA (Learning and Inference with Schemas and Analogies) was developed in our lab in collaboration with John Hummel. The LISA model has been used to simulate both normal human thinking and its breakdown in cases of brain damage.
Current research in collaboration with Hongjing Lu is directed at the problem of learning relations from non-relational inputs (e.g., word embeddings created by machine learning), and then using distributed representations of concepts and relations to find analogical mappings. The core computational models created in this project are BART (Bayesian Analogy with Relational Transformations) and PAM (Probabilistic Analogical Mapping).
CAUSAL INDUCTION
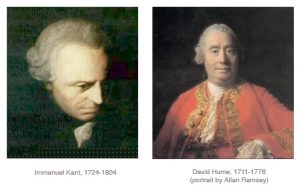
Historically, causality has been the domain of philosophers, from Aristotle through to Hume and Kant. The fundamental challenge since Hume is that causality per se is not directly in the input. Nothing in the evidence available to our sensory system can ensure someone of a causal relation between, say, flicking a switch and the hallway light turning on. Causal knowledge has to emerge from non-causal input. Yet, we regularly and routinely have strong convictions about causality.
Cheng and her colleagues have developed a theory of how people (and non-human animals) discover new causal relations. Her power PC theory (short for a causal power theory of the probabilistic contrast model) starts with the Humean constraint that causality can only be inferred, using observable evidence as input to the reasoning process. She combines that constraint with Kant’s postulate that reasoners have a priori notions that types of causal relations exist in the universe. This unification can best be illustrated with an analogy. According to Cheng, the relation between a causal relation and a covariation is like the relation between a scientific theory and a model. Scientists postulate theories (involving unobservable entities) to explain models (i.e. observed regularities or laws); the kinetic theory of gases, for instance, is used to explain Boyle’s law. Likewise, a causal relation is the unobservable entity that reasoners strive to infer in order to explain observable regularities between events.
The power PC theory explains many phenomena observed in both causal reasoning studies with humans and classical conditioning experiments with animals. The theory provides a unified account of a variety of types of causal judgments. In recent work the theory has been integrated with Bayesian inference to extend its applicability to a wider range of data.